I should emphasise that Generative AI is one of many tools that insurers can leverage to help achieve corporate goals. GenAI will not ensure an insurer will set the right vision, goals, and strategies, or allocate the optimal resources to be successful. And there are more AI tools in the technology toolbox.
The hard visionary graft to achieve competitive advantage remains the task of the C-Suite and leaders across the enterprise who must harness the strengths and tackle the weaknesses of the new kid-on-the-block, GenAI.
This article draws on an EY Parthenon study (see Further Reading for full report link) which shows that insurers are adopting GenAI for a variety of goals and discusses the pros and cons of each before concluding that insurers should not tackle just one goal at a time. I have added a fourth goal- in fact, it is vital to plan and achieve that before being able to achieve goals 1 to 3.
But let me add a caveats. Insurers should leverage all branches of AI as Gartner recently stated: -
‘By the end of 2024, value will be largely derived from projects based on familiar AI techniques, either stand-alone or in combination with GenAI, that have standardized processes to aid implementation. Rather than focusing solely on GenAI, AI leaders should look to composite AI techniques that combine approaches from innovations at all stages of the Hype Cycle.’ In fact Gartner states that GenerativeAI has reached the point of Inflated Expectations and likely to dip down into the trough of disullusionment before enterprises learn how to where to utlise it effectively.
Goals for Composite AI
- Productivity improvement and cost reduction
- Increased revenue and profit
- Competitive differentiation and advantage
- Data maturity to create a universal data layer
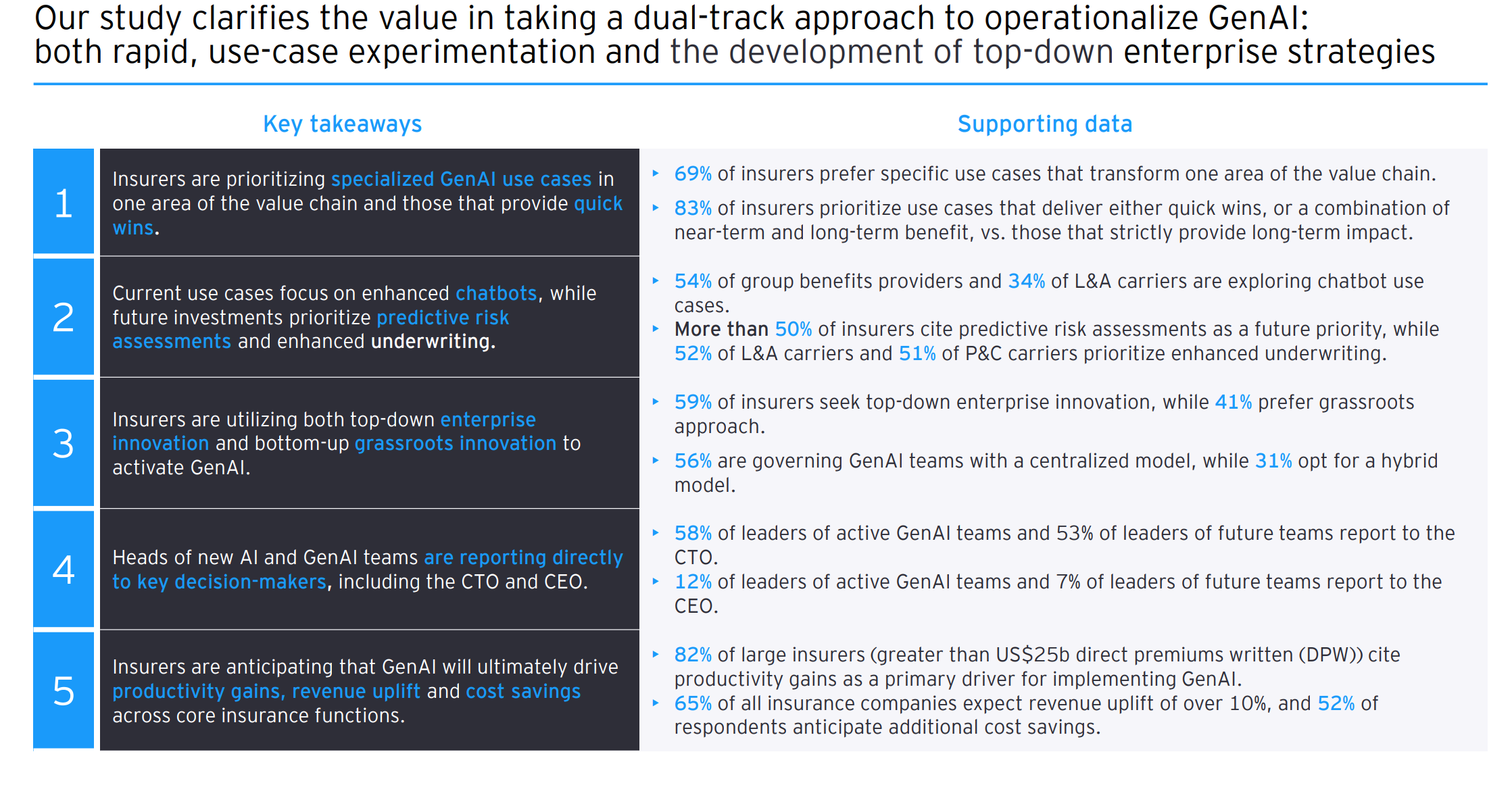
Source EY Report Generative AI in Insurance May 2024
Lastly, we will review one challenge that must be tackled before GenAI can be leveraged effectively. Markedly improving data quality and relevance. Many insurers seem to feel that they can leapfrog this hurdle.
Productivity improvement and cost reduction
Follow the money and you'll see that coding is the current ‘killer’ use case for GenAI deployment today. Productivity improvement is the key goal which itself leads to reducing costs.
“A McKinsey analysis from last year found that the direct impact of AI on the productivity of software engineering could range from 20 to 45 per cent of current annual spending on the function, with benefits including generating initial code drafts, code correction and refactoring. “By accelerating the coding process, generative AI could push the skill sets and capabilities needed in software engineering toward code and architecture design,” McKinsey said.”
FT August 23rd, 2024
It is the grunt work that GenAI tackles; skilled and experienced 'humans in the loop’ remain vital.
“No GenAI knows about good software architecture, or how to put systems together,” he added. “That’s still the thing we have to think through ourselves.” Marc Tuscher is a deep learning scientist and CTO of Sereact, a German robotics start-up.
It is not feasible to expect GenAI coding to deliver enterprise software. The code bases of these are just too large. There is insufficient token capacity in even the largest LLM models to cope. For smaller apps and partial rewrites, these GenAI tools are useful though remember that the output will rarely be above average quality, It will likely include errors, and you must always be aware of IP infringement.
That's a yes then for productivity gains with parallel cost savings which are also a main driver for insurers’ call centres with large numbers of agents.
A Stanford and MIT study found that call centres (all industries and not just insurance) were 14% more productive when using AI conversational assistance. On the other hand, the study showed a little-discussed fact- GenAI and large language models trained on massive data sets will by definition only predict average or slightly above-average outcomes.
McKinsey states that inexperienced and lower-skilled workers augmented by Gen AI gained a 35% productivity increase. Higher skilled and knowledgeable agents? Little or no improvement. So, expect to increase the quality outputs of the bottom half of agents but not to achieve industry-high customer satisfaction ratings. Productivity gains and cost reduction are the deliverables.
What about AgenticAI which is promised to transform all areas? This employs reasoning processes to self-learn and is embodied in OpenAI's O1 nicknamed strawberry to spend more time reasoning, test alternative predictive models and choose the best outcome. The key question is this. Is it prediction based on past outcomes rather than actual thinking? Yes- And any errors, which we know LLMs are liable to deliver, once adopted will be perpetuated in future models.
Microsoft has launched its next phase of CoPilot Wave2 and Salesforce added AgentForce to its toolbox. CoPilot has that PC-centric approach that has benefitted Microsoft for decades. Combining web data with a person’s own data, and that of the organisation. It does have a similar weakness to the attraction of Excel for accountants, underwriters and actuaries, project managers, and business managers.
The ability to use flexibly outside the constraints of company central IT and finance. The end-result of spreadsheets was anarchy and the gradual corruption of complex spreadsheets that yielded error-strewn outcomes. The same can be said of PowerBI, and all the analytics software loved by LOB staff.
Automation, self-learning, and reasoning AI incorporated in AgenticAI has all the inherent dangers of democratised spreadsheets and analytics software i.e. anarchy and error.
That again highlights the necessity for human oversight to battle the danger that is embedded in the very essence of AgenticAI- automated, real-time training and testing.
The lesson- use the services of people/technology partners who know the pros and cons of all types of AI. Not just GenAI but extractive and conversational AI for example.
The big tech companies and AI startups, connected by investments by the former in the latter, may be said to have a self-interest in promoting GenAi and LLMs as such deployments increase the demand for data centres, cloud computing, and services the former are investing eye-watering sums in.
That describes the deployment of LLMs trained on massive amounts of data to deliver generic models. The opposite end of the scale is the use of small language models trained on proprietary data and enhanced with the domain experience of professionals across different parts of the insurance value chain.
EY Parthenon found that 82% of large insurers are concentrating on productivity as the main driver to deploy GenAI. Furthermore, 69% have chosen specific use cases, and 83% quick wins. That seems to point to proprietary data-trained SLMs rather than generic LLM models to achieve these goals. Or LLMs trained generically and then fine-tuned on proprietary data.
And what are the other goals set for GenAI deployments in the EY survey?
- 65% of insurers set a revenue increase of 10% as a key goal.
- 52% set cost savings as a goal.
Is that because that is a long-term project and insurers want quick wins? Or is that a current limitation of GenAI? Despite what vendors say, GenAI does not think or reason. It uses brute computing power to see what tokens, typically words, follow the previous one in the massive data sets they are trained on to predict the next one. GenAI creates a credible-looking output whether words, computer code, images, sound, or false identities and hard-to-detect fraud. But that includes errors, hallucinations, and average level outputs that the smart presentation layers can hide.
Increasing revenues and profits
I see one problem with the majority goal of increasing revenues by 10%. Unless industry GWP growth is 10% not all insurers can achieve that goal. For top performers to deliver on that target others must stay flat, and a large proportion suffer declining revenues.
Being able to manage distribution more efficiently, effectively, and faster will help achieve growth. The insurers with a well-placed submission placed first in the queue, with the cover required and an attractive price is more likely to win the business than slower submissions.
This is not just a matter of sales but also operational and administrative systems as complex as those used by carriers. There is a need for real-time administration, transaction processing, and robust corporate infrastructure. This foundational understanding is key to harnessing the true power of GenAI to unblock unstructured data and incorporate it into decision-making.
Profitability demands better underwriting and pricing of risk. Underwriters spend almost half their time on admin, trying to make sense of spreadsheets rather than contributing to the insurers’ growth and underwriting profit. See further reading below for a full article on what makes for trailblazing underwriting performance.
Winning a bigger share of the industry GWP pie at a better profit margin will naturally make a big difference to competitive standing. But just doing the same things better will eventually run out of runway. In a changing world with unmet demand, and challenges like climate change, can GenAI help achieve competitive advantage and differentiation?
Competitive differentiation and advantage
Strategic and creative analysis, thinking and predicting the future are strengths of us homo sapiens. Our brains, creativity, powers of reasoning, and capability of lateral thinking. Freeing up time by productivity gains to apply these strengths and test new ideas can lead to differentiation.
Joe Reisberg CIO at US insurer EMC wrote about this in HBR back in April 2024.
What was the challenge?
Our agents’ relationships with customers are crucial, and we wanted to brainstorm ways to further improve them. We fed ChatGPT a few key documents about our company and its services. Then we asked our agents to find the most creative answer to this question: “How might we develop new ways to optimize interactions to enhance agent relationships and ultimately deliver superior customer service?”
Were you surprised by the results?
I was! When I left work that day, I thought that the quality, quantity, and depth of the answers ChatGPT had given my employees on the experimental team were really powerful—surely better than the human-only answers. Turns out I was wrong.
How so?
My team came up with four or five ideas, fed them into the AI system, and asked it to improve on them. Once the system had generated a few responses, they accepted them without asking it for more-nuanced or -creative ones; they were so sure it had given us the best results right off the bat. But the system had generated what it thought would be the “right” solutions—the most logical ones available with the information it had—whereas my colleagues had been tasked with finding the most-creative ones. Too often the AI-assisted teams defaulted to just pasting ChatGPT’s sensible but generic answers into a Word document.
What lessons did Reisberg apply to rectify that?
working with ChatGPT requires direct, unsparing feedback. The more you question generative AI, the better its answers will be. Drawing on it after the initial round of brainstorming can help get people over creative hurdles. And if they’re able to repeatedly challenge the AI to improve its suggestions, they’ll come up with incredible material. It can be hard to learn how to tease out those answers, though; people need time and practice. And the immediate efficiencies from the technology probably won’t be as impressive as people hope. But the improvements from iterating with it—speed, productivity, creativity—will be tremendous in the long term.
The human insight and detailed prompting are vital. Do not expect to automate creative thinking. Augment your best people.
Beware an inherent danger that the very strengths of GenAI will shorten any advantage gained though.
Competitors can use GenAI in the same way to develop similar competitive advantage. You might think that using proprietary data sets will protect you from that diffusion of new ideas. But such data is prone to leakage; people move companies and what’s to say your competitors do not have similar data. Especially in personal lines where customer churn is high, and similar customer data are generated across the industry.
My conclusion is that to achieve competitive advantage, insurers must have it in the first place. The top decile of insurers tends to feature the same names year after year. They already have a competitive advantage and successful GenAI deployment will help maintain that lead.
To leverage GenAI it must be used to embrace and improve your entire business model. But most insurers are only looking at short-term wins and individual use cases rather than the whole business. The EY Parthenon study shows that insurers intend to deploy across various use cases eventually: -
- At least 40% of insurers expect moderate to no human involvement across sales, underwriting, policy servicing, claims and back office in the next 10 years.
- Insurers anticipate an even larger impact on back-office and policy servicing, with over 55% predicting limited to no human involvement in the next 10 years.
- Of the 23% of insurers that expect very limited to no human involvement in back-office support within 10 years, approximately 50% have less than US$1b DPW, indicating both larger and smaller carriers expect impact in this space.
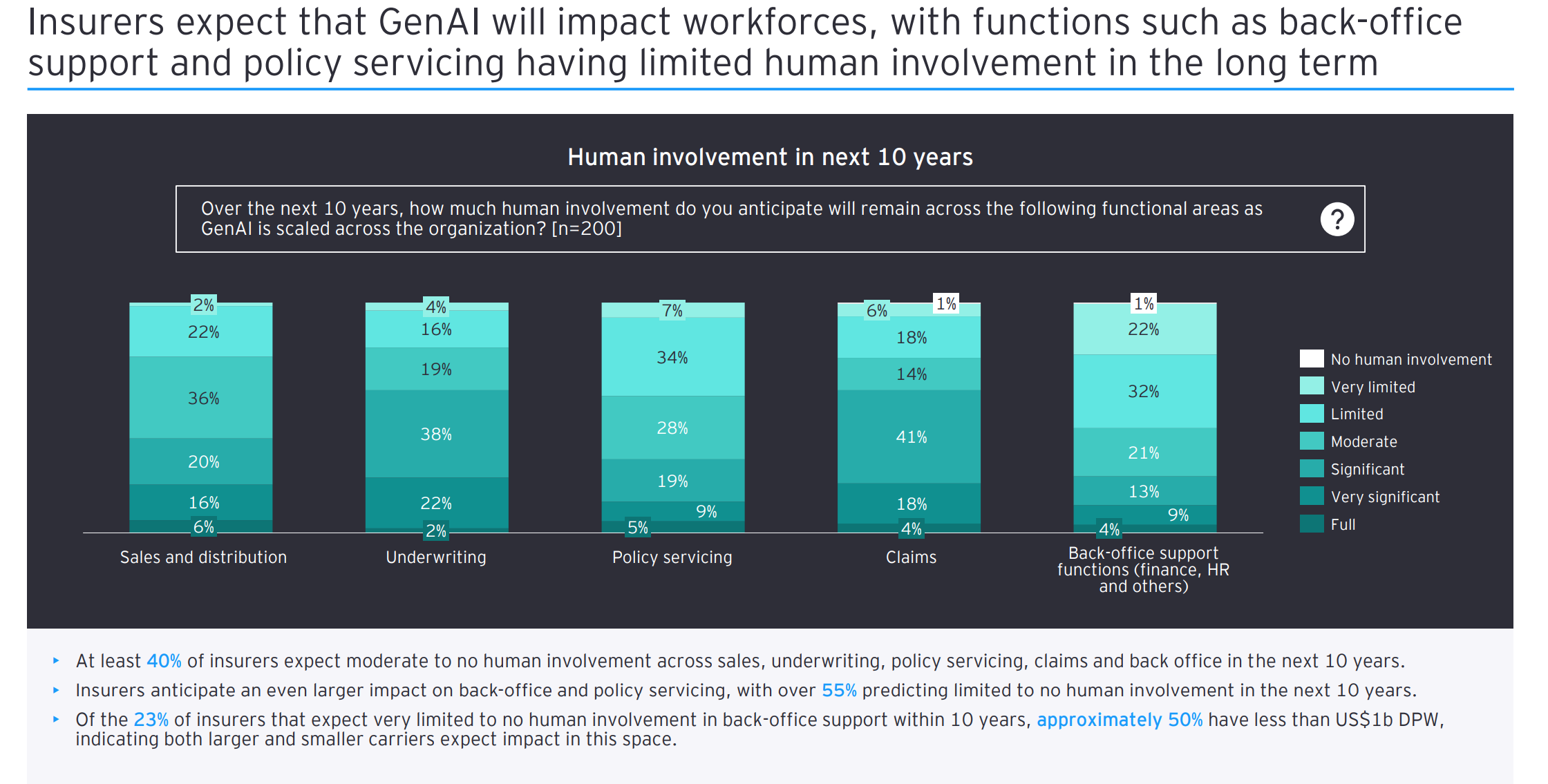
This productivity and cost-saving approach is expected to take years, if not a decade. But in a decade the continual development of GenAI and new tools could make ideation and creative differentiation more practical. Insurers really need to address the whole business model as well as individual use cases now or they will be left behind by those that apply that strategy. That requires the help of technology partners who know what they are doing. Across all types of AI, not just GenAI.
The current front-runners may be outmanoeuvred by more agile AI vendors with viable cost structures. Better hedge your bets!
Insurers also need technology partners with a particular expertise in data management. Able to combine your domain expertise with their tools and bridge the gap that all insurers must hurdle- hidden and unusable data most of it unstructured and in data silos. Without that, GenAI is of limited use. It will be like training world-class athletes on Big Macs and Southern Fried Chicken rather than balanced nutritional diets.
I rather like this quote from the CTO of AI specialist Palantir.
Data maturity and a universal data layer
Shyam Sankar: “I think the critical part of it was really realizing that we had built the original product presupposing that our customers had data integrated, that we could focus on the analytics that came subsequent to having your data integrated. I feel like that founding trauma was realizing that actually everyone claims that their data is integrated, but it is a complete mess and that actually the much more interesting and valuable part of our business was developing technologies that allowed us to productize data integration, instead of having it be like a five-year never-ending consulting project so that we could do the thing we started our business to do.”
I suggest that most insurers embrace GenAI without having the required integrated universal data layer.
Palantir is not the only one tackling this issue. Aiimi Ltd has honed its skills on blue-chip enterprises and like Palantir, entered the insurance market.
Aiimi offers an ‘Insight Engine’ that can find, categorize and label unstructured data hidden in data silos across the enterprise, creating one universal information layer. Applying term matching and contextual awareness it shrinks this to the relevant and real-time data required by both employees and AI- departmental and enterprise needs.
Not just for one part of the value-chain but the whole business model. A substantial benefit is that you do NOT have to integrate/centralise the data but can leave it in the original sources and still feed the AI platforms and apps.
Insurers need this category of partners to operationalise GenAI, apply it across the whole business model, and come closer to achieving a sustainable competitive advantage.
Another toolbox and not a solution
AI was first deployed in the mid-20th Century so it is not new. The current set of GenAI tools has been developed using the vastly increased power of hardware, specialist chips, and ecosystem to apply brute force to deliver outputs faster.
Most new technology projects fail to meet their original objectives and there is no reason to believe GenAI will be any different. To be in the trailbazing top decile, insurers must plan and resource the deployment of technologies to support staff and partners across all the value chain. They must get the buy-in of all participants and ensure they have the training to leverage these new tools. GenAI will otherwise amplify all the weaknesses and faults of the current organisation and add a considerable cost.
Further Reading
GenarativeAI in Insurance by EY
AI Won’t Give You a New Sustainable Advantage in HBR
Don’t Let Gen AI Limit Your Team’s Creativity in HBR
Surveys highlight data maturity holding back AI deployment ambitions
Underwriting trailblazers outgun mainstream insurers
For organizations and their employees, this looming shift has massive implications. In the future many of us will find that our professional success depends on our ability to elicit the best possible output from large language models (LLMs) like ChatGPT—and to learn and grow along with them. To excel in this new era of AI-human collaboration, most people will need one or more of what we call “fusion skills”—intelligent interrogation, judgment integration, and reciprocal apprenticing.
