I attended a really useful briefing by Procurato recently aimed at helping the C-Suite and LOB Managers navigate the tidal wave of innovation leveraging AI. Helpfully, they published their insights yesterday and gave me permission to publish these.
AI is neither a new toolset nor has it really had a revolutionary impact across insurance. Innovation takes longer than expected, and the impact tends to be over-estimated in the short to medium term and under-estimated in the longer term by which time industry leaders will be leaving competitors in their wake. Swiss Re has a valuable visualisation of where AI will have the most impact for insurers.
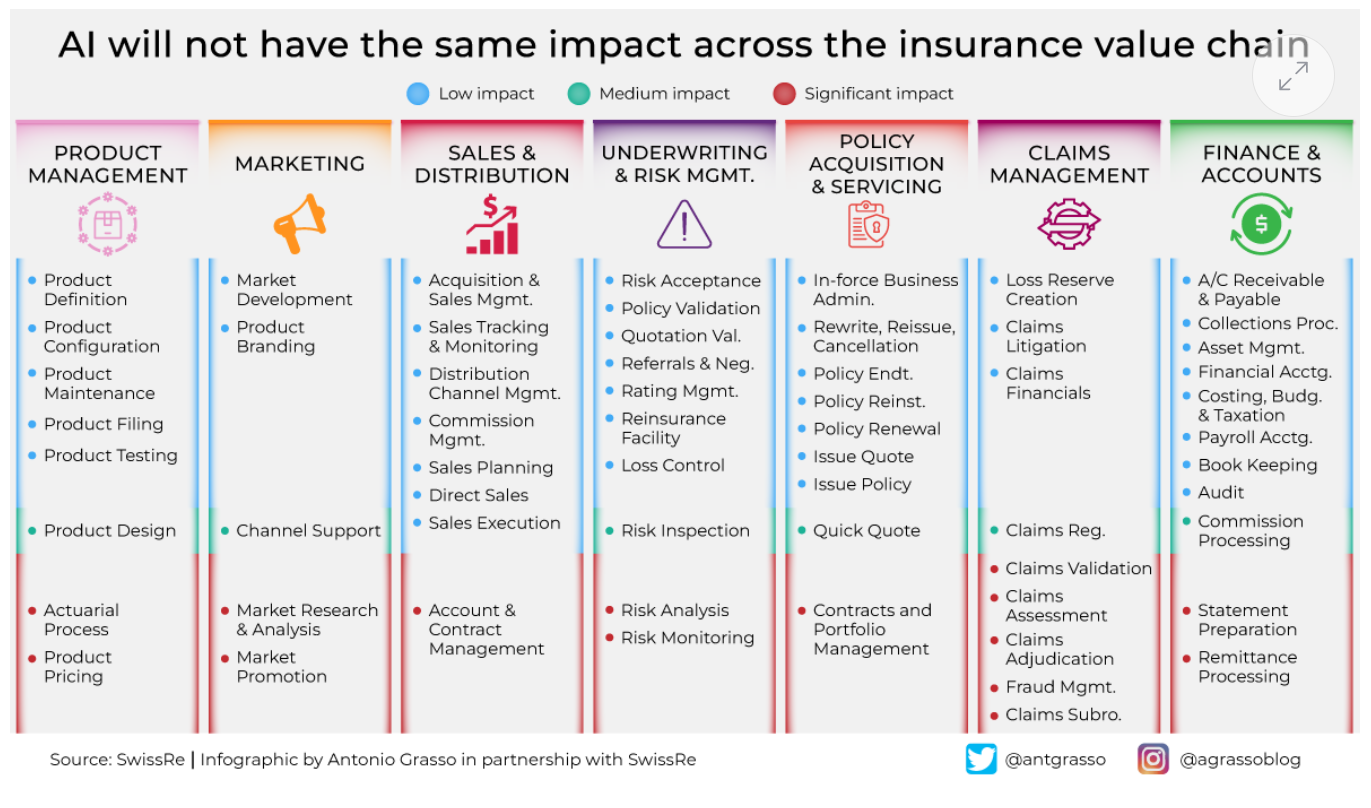
The first section of this article on how to navigate the picture above is followed by a call to action inspired by a major article in The Harvard Business Magazine: "Stop tinkering with AI and get stuck in!" with plenty of practical advice as to how to plan, organise, and deliver the outcomes desired.
First Navigating AI usage by Procurato
“Have you heard about ChatGPT? It’s amazing! It can generate any type of content about any subject after just a few instructions, you’ll LOVE it!”
“Why would I love something that overrides the years of experience in my profession, and will no doubt see me out of a job?”
This exchange is a common conversation that is happening across the world in relation to AI’s hottest new tool. It appears everyone has a love or hate opinion.
While ChatGPT is generating extensive amounts of media coverage (and Google’s launch of Bard is sure to increase the chatter) and public opinion let’s not forget we’ve been surrounded by AI for years. Remember when we started noticing how social channels such as Instagram started filling our personal feeds with images and videos because we watched a five-second clip of a cute dog? Not an issue when you like looking at images of cute dogs, much more of one when you only watched the first one by accident and have a fear of them.
Whether you personally ‘opt in’ to the benefits of AI or not, there’s no doubt it has a big influence in our lives. All ‘it’ wants to do is make our lives a little easier and help give us more of what we want, or what it thinks we want (depending on which way you look at it.)
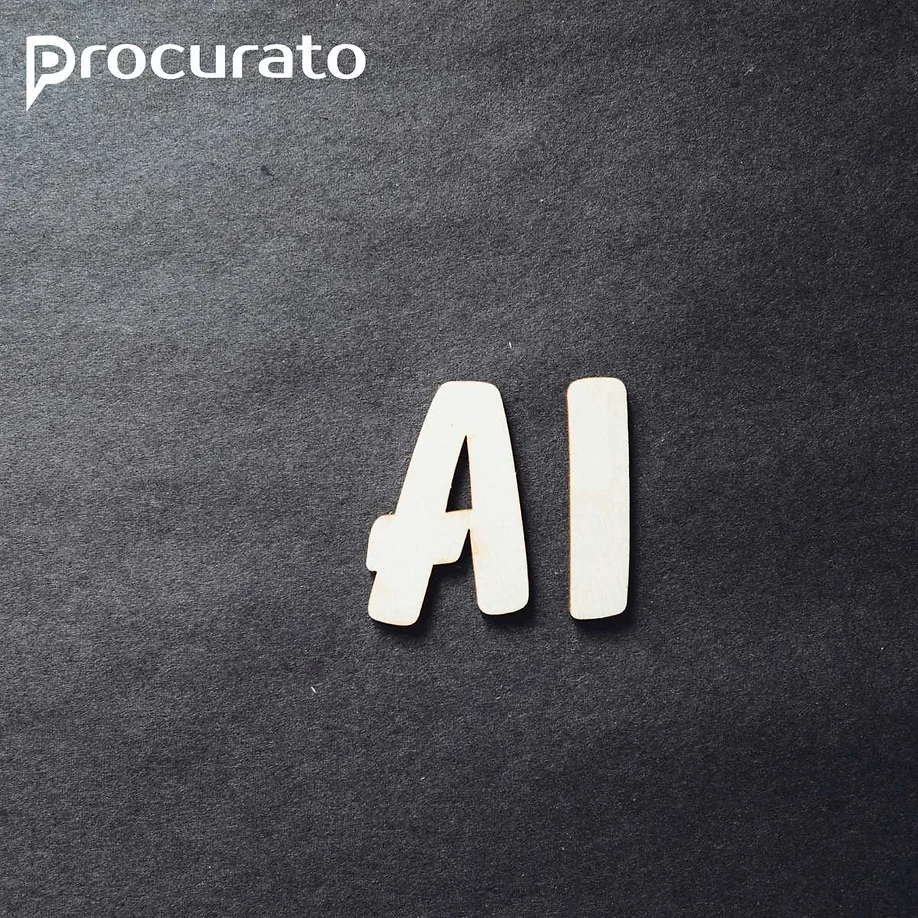
Image Source: Unsplash
- Reducing human error
- Automating manual skills and processes
- Applying known data in new ways
- Learning new data and finding patterns in behaviour
- Streamlining processes
From Chatbots to risk assessment….
Back in the late 1990s / early 2000s GPS became commonplace, and that advance made telematics possible. Insurers and brokers believed it was the technology of the future, a ‘black box’ for drivers encouraging them to drive more safely and be rewarded for it. A tool to provide added-value concierge services to help manage the activities of fleets and essential-use drivers. It didn’t and, to a great extent, still hasn’t had the predicted impact 25 years on. However, the continuing advance of technology means it’s now not only about keeping to the speed limit and taking a few pounds off a premium. Consumers want more personalised offerings, behavioural-based telematics if you will. And insurers can and are adapting to that. The point is, that what was once the darling of insurance technology is now a little passe; the world has moved on and insurers need to move with it. But in the background, for those promoting and using telematics, artificial intelligence is very much at the core of the product – the algorithms are churning the collective and individual data to ‘recommend’ a driver score, premium, course of action – to nudge the driver, to warn the underwriter.
This example is just one of how AI has been used in the insurance sector over the last few decades and as technology continues to advance so does its uses.
Underwriting
In years to come will personal lines underwriting operate in the way it does now? According to McKinsey’s Insurance 2030: The impact of AI on the future of insurance report ( see link at bottom of article):
Agents use smart personal assistants to optimize their tasks as well as AI-enabled bots to find potential deals for clients. These tools help agents to support a substantially larger client base while making customer interactions (a mix of in-person, virtual, and digital) shorter and more meaningful, given that each interaction will be tailored to the exact current and future needs of each individual client(1).”
They’re bold statements but in our opinion the likelihood for them to come true is high. Insurers first used machine leaning (ML) for underwriting in the 1980s. ML’s ability to make predictions using customer data started to revolutionise insurance risk models. But it would be around 25 years later before AI came into its own in UK financial services, most notably enabled by the rise of aggregator services.
In the UK, consumers were quick to embrace the use of aggregator channels and by 2009, they accounted for more than half of total private auto insurance sales in the UK and 36 percent of home insurance sales(3). Once deemed as a potential challenge for insurers this approach is now firmly embedded in the omnichannel experience. Customers benefit from the level playing field and ease of transaction whereas insurers benefit from much bigger reach of potential new customers and better conversion rates that ultimately can reduce acquisition costs.
We have previously talked about labour shortages and fewer people entering the underwriting profession. AI addresses these challenges when underwriting less-complex policies. Insurers hold a vast amount of information on their systems about individual policyholders and the use of AI enables them to create risk profiles which allows the purchase of personal lines policies to be completed in a short space of time. In addition, extras can be added on at the point of purchase further adding data to that profile.
It’s also important to recognise here there are a number of terms – Big Data, Artificial Intelligence, Machine Learning, Predictive Analytics – which are often casually interchanged, when they are quite different things. All share a simple core principle, most precisely articulated in Bill Gates’s recent seven-page ‘letter’, “The Age of AI has Begun(11)”:
“Compared to a computer, our brains operate at a snail’s pace: An electrical signal in the brain moves at 1/100,000th the speed of the signal in a silicon chip! Once developers can generalize a learning algorithm and run it at the speed of a computer—an accomplishment that could be a decade away or a century away—we’ll have an incredibly powerful AGI. It will be able to do everything that a human brain can, but without any practical limits on the size of its memory or the speed at which it operates. This will be a profound change.”
The key point being that we’re not talking about replacing workers with automation, we’re talking about capability already in existence which can do things people would never, realistically, be able to do. The AI already in play today has made a leap equivalent to the difference between horseback and air travel.
Risk and the claims process
The ultimate beauty of AI is its power of insight. Insurers already capture a significant amount of data – some argue they are more akin to knowledge-management businesses than financial institutions. They know their customers’ demographics; they know if they live in an area with high levels or crime or a higher risk of flood risk. They know their customers’ claims history; they know whether the jobs they do may have an impact on their policy. They know that someone who has a dog may be less likely to be burgled but could be more likely to have accidental damage.
There is so much more that AI can bring to the table. With its power to analyse multiple data points in the complex real-world AI models are able to do a better job of predicting outcomes. As well as the structured data that comes from insurers’ in-house systems AI can also analyse unstructured data which we class as those that don’t fit neatly in the system fields. This unstructured data could include emails, video files, audio and dash cam footage. Assuming that the models are built to understand the data (see AI challenges later in this article) AI can prove invaluable to predicting insurance risk.
There is no doubt that being able to predict insurance risk will improve decision making.
It can also boost productivity through more efficient modelling and these models should reduce the frequency and severity of losses which, in today’s competitive aggregator market, is where organisations are going to achieve financial advantage.
Protection from financial loss
In our recent update on home inflation we advised that insurers are telling us that fraudulent claims are on the rise. While there is no indication that policyholders are deliberately damaging their possessions there is data to suggest the value of the claim is exaggerated. This is not a new trend.
Aviva reported 11,000 instances of claims fraud worth more than £122m in 2021 and at the time of writing their report, 16,700 claims were under investigation for fraud(4). Whiplash fraudsters are switching to vehicle repair claims and homeowners faced with a cost-of-living crisis are exaggerating their claims.
AI is increasingly being used to help insurers detect and prevent fraud. From analysing social media and scanning images to reviewing data from previous claims AI can analyse data to flag a potential fraudulent claim giving call centre operatives an opportunity to probe further and may even adjust pricing for future policies.
Natural disasters – past experience is not the predictor
In April 2022 the ABI announced that insurers could expect to pay out nearly £500 million to support customers hit by damage from Storms Dudley, Eunice and Franklin(6). Later in the year subsidence claims started to increase following the unprecedented hot weather spell in the UK. Climate and natural disasters continue to have an impact, and this isn’t going away any time soon.
Unlike many other risks historic data isn’t often a good predictor for climate events. Who could have predicted 40-degree temperatures in the UK on the back of those storms earlier in the year? Climate change is altering the world’s weather patterns and to combat this many insurers are turning to InsurTech firms to find alternative solutions to better predict the weather and model the risk accordingly. According to PWC’s State of Climate Tech 2022 report climate tech funding in 2022 represented more than a quarter of every venture dollar invested that year7 so it’s being seen as a priority by the big players.
Thanks to the predictive qualities that AI and machine learning has, we are seeing much improved models to predict natural disaster events. According to TechTarget(8) ‘traditional physics-based models are still outperforming AI models, but they operate at tremendous computational cost that AI models can mitigate when used correctly.’ They also cite AI is already being used to prepare for disasters using aerial imagery technology, location data and satellite imagery to look at building and roof characteristics, surface permeability, roof condition and nearby construction and building footprints. This technology allows users to see ‘predictive insights like levels of tree cover in proximity to buildings that could result in increased vulnerabilities caused by fire spread or damage from fallen trees.’
These models can analyse building conditions over time to see if for example a roof can withstand a weather event or if it should be replaced. Being able to predict potential damage before it happens could be beneficial to insurers who can add this as extra coverage when underwriting a policy. Although parametric solutions are already well-established insurers should continue to explore emerging technologies and scale them because as climate-related events increase so will the pay outs for customers and clients.
However, insurers may do well to consider the recent technology developed by the Met Office and Bristol University. They’ve developed climate prediction and flood models which they are ‘100 percent certain’ where and when heavy rainfall will occur. It’s a ground-breaking flood model and combines historical risk and events while combining them with climate model projections. These predictions have been analysed against ABI flood loss data and hold up well. As insurers will know, where there is certainty, there’s no risk so who would underwrite a location that is guaranteed to make a loss? To use an analogy, it’s the equivalent of insuring a vehicle you know is going to crash. Insurers will have tricky decisions to take to support customers in the face of technology such as this.
A step past telematics
The so called ‘In home’ Internet of Things (IoT) brings many opportunities to insurers through personalisation, improved risk predictions and prevention.
In a similar way to how insurers offer telematics solutions to drivers they can offer connected devices for the home which can monitor its security and safety. Should an issue arise the IoT system would alert the insurer which would allow them to step in to prevent that damage, providing a safer home for the customer and less risk for the insurer. Traditionally insurers do provide better premiums to policyholders who have better security of their home and vehicles but if this were to be expanded not only would customers benefit from cheaper policies they’d feel more protected by their insurer.
We’re already seeing that the cost of IoT devices is falling as their use become more widespread. If insurers were to offer the device in conjunction with their policy this could be seen as a competitive advantage and will lead to fewer claims.
As with so many of these “opportunities”, one of the challenges for insurers is to have enough time and expert resources to know what to do with them. It takes a lot of thinking, which is exactly why insurers should partner with new technologists.
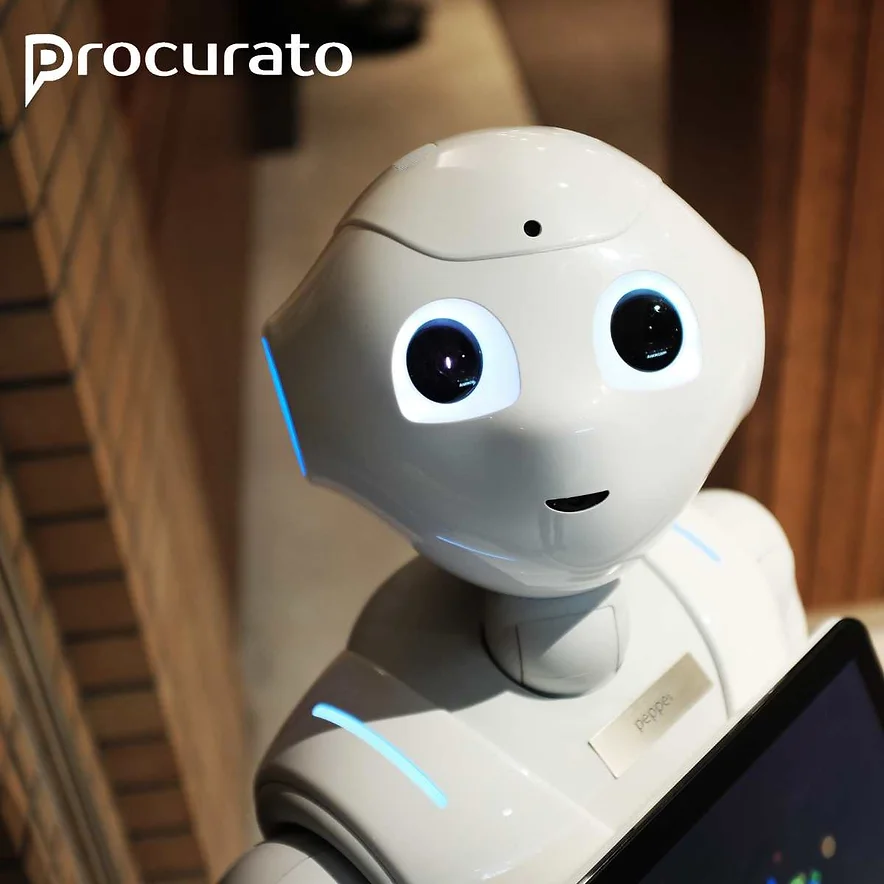
Image Source: Unsplash
Customers conversing with bots
One of the most common uses of AI in the insurance sector are chatbots. It’s not uncommon on any website for a chat box to appear with a chat bot asking if they can help. It’s an easy technique to reach a customer or potential customer within seconds of them reaching your page; you don’t want lurkers you want sales.
While 60 per cent of consumers say they prefer to speak to a real person when dealing with a query5 a significant number are happy to speak to a bot when they need a simple question answered. Bots are a perfect solution to give answers to simple, non-urgent requests and to provide them out of office hours. Insurers have been using chatbots for considerable time; it’s freeing up the time of customer service representatives who answer many similar FAQs every day. But what else can a chat bot do in the insurance world?
- Automatically process claims
- Explain insurance plans in simple terms
- Provide cover policy details if lost.
- Automatically qualify customers.
- Assist customers with insurance payments.
- Receive feedback on plans and customer service while simultaneously measuring the sentiment of online discussions which can provide ongoing feedback.
- 24/7 availability reducing the time needed in call centres.
Further support for contact centres
Aside from ChatBots AI offers many more benefits in call centres. Research from Qualtrics(9) has indicated that 63% of consumers said companies need to get better at listening to their feedback with 60% saying they would buy more if businesses treated them better. AI can enable this by:
· Understanding a customer’s full history at the touch of a button: Customers expect their insurers to have access to all their previous correspondence and don’t expect to have to repeat themselves to multiple agents. AI can scour huge amounts of data to bring together one version of the customer truth.
· Providing empathy through natural language processing: Some AI solutions have the ability to understand voice and text even if the customer is not speaking in their first language. This enables call centre staff to understand the emotions of that customer and can even provide suggested commentary. This along with understanding a customer’s history can be further enhanced via the provision of personalised real-time scripts for the call handler.
· Providing quicker responses: Customers can be frustrated when they don’t hear back in suitable time on their correspondence. AI can enable a receipt notification and subsequent ones such as being read, understood and who it has been re-routed to.
In addition, AI can provide business support in the contact centres through:
· Automating coaching and quality management AI in the call centre can automatically track agent performance, successful resolutions, and script compliance. With that information, AI-powered contact centre software suites can offer up real-time insights on exceptional performers and where there are coaching opportunities.
· Providing new insight. With AI tracking 100% of all customer and agent interactions it provides even more insight which can benefit the business. This could be through determining new trends or requirements or by highlighting any issues.
· Improving workload and reducing employee burnout. If AI can take away the administrative and less complex tasks away from employees it will free up their time to focus on important tasks that add benefits. This in turn is also likely to improve employee satisfaction.
· Optimising supplier relationships. One client we have spoken to has shared their experience of testing AI is to better support opportunities for salvage through improved keyword use and searches extracted from insurance reports which should identify more accurate salvage detail and prospects. This improves responses and creates less unnecessary land fill.
Procurato recently conducted a piece of original research on behalf of a major Insurer client who wanted to understand what their broker customers actually wanted. Over five months we reviewed the market, interviewed many brokers, talked to other industry experts and the outputs were reassuringly straightforward:
- Keep it simple and make it easy for me to use i.e., authentication, interface, recording of chats.
- If you’re going to do chat, make sure it works for me, and it doesn’t just put me in a different queue from the phone queue you’re replacing it with.
- Make your digital engagement do what I’ve been asking you to do for years; if you give me bells and whistles but I can’t access the data I need, send the files I want, or update the records I’m working on, I won’t use it.
AI in insurance – challenges
It’s clear to see there are many benefits to implementing AI in whichever form to a business but with so much available it’s important to be able to cut through what is potentially ‘hype’ and what is really needed to achieve an organisational strategy.
A challenge we often hear from CFOs is the pressure to balance the significant investment of these (often still) emerging AI technologies against the potential payback. Many technologies have not been proven and insurers must decide whether to get ahead of their competitors or risk poor spending decisions.
Investment is just one issue, there are other considerations when choosing an AI tool:
· Aging IT systems can stand in the way of delivering digital solutions for its customers and employees meaning investment is not just about the new tech but the existing.
· Bias and Ethics. Analytical models need to be built and ‘trained’ to find relevant data. If it doesn’t this can lead to biased predictions or outcomes particularly if the models learn from human-labelled data. Most of the regulation in place today for insurers is founded on fairness, which needs to be evidenced transparently and there needs to be accountability for data bias. Understandably, those personally regulated individuals are nervous about entrusting their reputations to computers.
· Weaknesses in the data AI understands. While AI / ML models can turn complex structured and unstructured data in actionable insight the data needs to be current, accurate and well maintained. If a model is built with poor quality data, you can expect to see poor quality outputs.
· Data security and fraud. Cybersecurity attacks can be more prevalent when accounts are linked. This could be in the form of connected devices which could put not only your business at risk but also your consumers. Additional care must also be taken when integrating with your customer database.
· Customer concerns: Some customers may be reluctant to share their personal data with insurers particularly when using connected devices. They will also want reassurance that their data is only used for the purposes that it’s intended for. Breaches of data privacy can lead to huge fines and loss of reputation for an organisation.
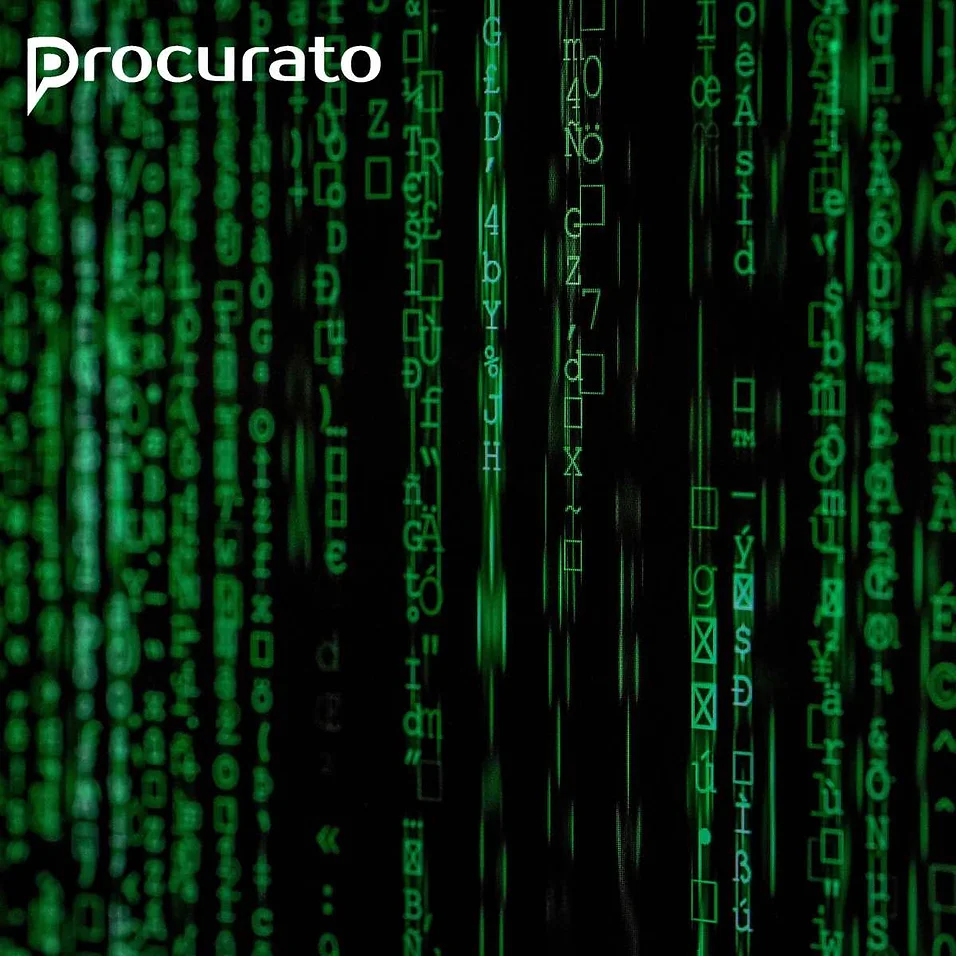
Image Source: Unsplash
Looking back on AI’s history it’s unlikely we’d ever predict where it is now compared to where it was when it started and the same applies if we look too far into the future. But we are seeing emerging technologies.
· The Metaverse. Consumers are already immersing themselves in the metaverse when buying property, cars, furniture, clothes and artwork. We have also seen the rise of digital properties such as non-fungible assets (NFT). All of the above have insurable value and insurers will need to adapt.
· ChatGPT and Bard. Still in their relative infancy for public use ChatGPT and Bard (Google’s version) is limited in some abilities such as handling multiple tasks and not being able to understand some human context such as colloquialisms or sarcasm but there are some potential uses. In addition to being able to simulate conversations or providing realistic helpful responses, both are designed to augment existing search facilities.
Roi Amir, CEO of Sprout.ai suggests that the technology could be used by insurers to start their own innovations, “[Chatb is a great generalised model. What I think we will see is many companies using it as their baseline model and add layers of intelligence and specificity for their individual domains(10).”
AI is continually evolving, and insurers should be willing to evolve with it. It brings tremendous benefits but not without its challenges, so why not speak to Procurato about the best solution for you.
Now the call to action; stop tinkering and get stuck in with plenty of practical advice.
Summary.
AI initiatives at many organizations are too small and too tentative. They never get to the only step that can add economic value—being deployed on a large scale. Testing the waters may deliver valuable insights, but it probably won’t be enough to achieve true transformation. A pilot program or experiment can take you only so far.
The proof is in this study by Accenture which shows the insurance industry lagging behind others.
Sound familiar? There are usually many hurdles in the way of getting beyond the initial micro steps; data silos, multiple unconnected technology stacks, and inflexible core technology. You name it! But the fact is some insurers are surmounting these obstacles. Read this practical advice but first, lets look at those hurdles in a bit more detail before looking at the solutions.
"At many organizations, AI initiatives are too small and too tentative; they never get to the only step that can add economic value—deploying a model on a large scale. In a 2019 survey conducted by MIT Sloan Management Review and Boston Consulting Group, seven out of 10 companies reported that their AI efforts had had minimal or no impact. The same survey showed that among the 90% of companies that had made some investment in AI, fewer than 40% had achieved business gains over the previous three years. That’s not surprising: A pilot program or an experiment can take you only so far."
Thomas H Davenport & Nitin Mittal HBR Jan 0Feb 2023
Not to mention the need to face constant failure in order to relearn fast, iterate, possibly change direction and last the course to eventual success. One example of this comes from a different field altogether; see - US Marines defeat Pentagon AI test by hiding in cardboard box
Scharre writes: "They parked the robot in the middle of a traffic circle and the Marines had to approach it undetected starting from a long distance away." You're already on his- some Marines hid in a cardboard box and got to the robot without the algorithms having the faintest idea.
Even Tesla with the most mileage, event, and contextual data of any auto OEM is finding it a tough nut to deliver autonomous vehicles. But, they will continue the intensive efforts, and the rewards for those auto manufacturers that perfect AVs will be immense.
As Daren Rudd Vice President of Consulting - Head of Insurance Business Technology Consulting at CGI UK says " We are very much in the learning-by-doing stage on how to get the best out of these tools. You need to be out there testing our different approaches, seeing what works or doesn’t, and not be afraid to fail (or learn fast)."
One feels that insurance carriers and brokers are at this tipping point between experimenting with AI-powered software like Tractable, Solera, Shift, FRISS, BAIL, SLVRCLD, and Om:nius etc, and leveraging the full strategic benefits of AI. The huge amounts of data generated by sensors, connected property, vehicles, plant & machinery, and telematics are the fuel to power AI potential. And many companies have developed mature applications solving real problems but they need to join together in a strategic plan.
Successful AI today tends to be "narrow AI"- tight use cases for very specific tasks.
Yet too often the single application merely fast-tracks the prediction or decision to the next buffer of human decision-making unless these point solutions are planned in the context of deploying at a large scale and across the whole enterprise.
One factor that has held insurers back has been either legacy systems or the inflexibility of current core systems of record. It just takes too long, costs too much, and swallows too many scarce human resources and talents. What's the answer?
The new generation of No-Code/Low-Code, API Smart, and Micro-Services architectured CoreTech and ClaimTech like EIS, ICE, Instanda, Genasys, Ignite, and RightIndem are ideal platforms to start the plan to "Go All In" with AI.
Insurers such as esure, Liberty Mutual, The AA, and Simply Health have re-platformed to enjoy the competitive advantages of agility, faster innovation, and the ability to deploy technology ecosystems.
With these agile and inrerconnectable platforms carriers and brokers are better able to test short-listed applications in A: B trials with production-level claims and integrate the different packages chosen, the multiple and critical data sources, and do so a step at a time towards the ultimate goal of leveraging AI and data to the full.
All is not lost for those committed to the last upgrade of the core systems of course. RightIndem has integrated with Guidewire and other current core systems not to mention the new generation. You can still work on planning and deploying AI with the older architecture platforms; it is just a degree harder.
HBR describes 10 steps to success.
- Know what you want to accomplish
- Work with an ecosystem of partners
- Master Analytics
- Create a modular, flexible architecture
- Integrate AI into existing workflows
- Building solutions across the organisation
- Create an AI governance and leadership function
- Develop and staff centres of excellence
- Invest continually
- Always seek new sources of data
1) HOW WHAT YOU WANT TO ACCOMPLISH
This is a critical factor in whatever innovation and transformation you want to achieve. Why, and what clear objectives to achieve the company goals e.g.
- Improve process and settlement speed
- Reduce indemnity and operational costs
- Personalised products and services
- Build communities of customers
HBR recommends one well-defined, overarching objective that is a guiding principle for AI adoption; eg achieving improved process and settlement speed across a global insurer will require developing a global data model. Start with your national ones but aim to eventually deploy a single data model to achieve global competitive advantage.
2) WORK WITH AN ECOSYSTEM OF PARTNERS
A company needs strong partnerships to succeed with AI. Take carrier esure in the UK with its goal to be "The Insurer of the Future". It's partners include: -
- EY- SI and implementation partner
- EIS- CoreTech partner
- Amazon AWS- Cloud Infrastructure & Services partner
- Amazon Connect- Omni-Channel Call Centre partner
- RightIndem- Digital Claims Partner
Naturally, esure has also specified AI-powered apps to be integrated into this new technology stack.
3) CREATING A MODULAR, FLEXIBLE ARCHITECTURE
This is a pre-requisite to effectively deploy data, analytics, and automation across an insurer and its brokers and supply chain partners. Many a claims transformation project has been stymied by the resistance of brokers to adopt rigid technologies deployed by carriers. Focus on real problems, simplicity, and getting buy-in from all parties becomes easier ( but it's still not easy) with modular architecture (MA).
MA makes it easier to extract key terms from legal documents, policies, invoices, medical reports, freeform text, and speech.
How many auto insurance carriers in the UK analyse the current, timely, and valuable insights from MOT data? Percayso Informs Kieren Fischer brings this to life in a recent article:
"Percayso obtains this data directly from the DVLA, Fisher said, which ultimately is a live system but what’s interesting to see is that while some insurers are already consuming the most basic MOT data, few are digging deeper into the wealth of insight it can yield.
To his mind, the real benefit is around understanding the condition of the vehicle. When insuring an asset, he said, understanding that asset as much as possible and how likely it is to be involved in a claim is what insurers should be doing. So, Percayso is taking it one step further and starting to examine the results of MOTs, looking for advisories and failures and categorising these into different tranches.
This enables Percayso to deliver an MOT ‘risk score’ based on the condition of the car and, crucially, its maintenance at the point-of-quote and at speed, he said. And be able to understand these trends on a vehicle-specific basis and deliver these insights in a meaningful, consumable way is an interesting proposition that is translating well across the insurance marketplace.
How willing are insurers to embrace new data variables?
Fisher and the team are seeing significant appetite from insurers to engage with new data variables, which he highlighted is unsurprising given that everybody is looking for the newest exciting data to give them an edge in today’s competitive market. Having access to these processed results on vehicle data is a key way for companies to stand out but it also allows them to price better and write better business."
With a modular and flexible architecture such as that chosen by esure, it is practical to embrace the data, applications, and platforms to leverage AI.
4) MASTER ANALYTICS
This requires a commitment to using data and analytics for most decisions embedding AI in products and services and conducting tasks and even completing entire processes in a more automated and intelligent way. Typically, most enterprises' biggest obstacle is acquiring, cleaning, and integrating the right data. Take the matter of broking and placing business from retail brokers in the US onto the London Markets. Data is typically a nightmare; the surfacing and normalization of unstructured data is a massive challenge and a priority to manage effectively before contemplating AI ventures.
"Greater Than" can analyse a carrier's claims and GPS data and model against its unique global database and states that its predictive risk AI can reduce the auto loss ratio by 10 to 15 percentage points. Which carrier wouldn't at least test this? Especially when UK carriers are currently reporting increasing loss ratios and worsening bottom-line results.
5) INTEGRATE AI INTO EXISTING WORKFLOWS
Inflexible work processes are as much of a deadweight as inflexible IT architectures. Working back from the customer ( policyholders, brokers, agents, and supply chain partners who are at liberty to work for you or not i.e. choose other insurers in a tight market) HBR recommends you choose which workflows are ripe for AI speed and intelligence. It is the workflows that generate enormous amounts of data and repetition that will benefit the most.
The claims handlers on the frontline will have an eye for and knowledge of processes that will benefit and how they can be explicitly improved. Again, modular and agile technology makes this a practical goal.
6) BUILD SOLUTIONS ACROSS THE ORGANISATION
Once you have tried, tested, and mastered AI across specific workflows, say accidental damage home contents claims with STP, you can become more aggressive across the organization and deploy it in motor insurance. Can algorithms applied in one process be applied across the company?
Take the data analytics mentioned above to predict risk and price risk in a granular fashion. Why not use the same algorithms used to predict risk to be utilised to claims volumes and plan claims resourcing i.e. number and skill profile of claims adjusters and strategy to outsource claims?
7) DEVELOP AND STAFF CENTRES OF EXCELLENCE
To go all in with AI will require sufficient funding and time and heads of AI and excellence that can evangelise and implement projects across the various business units. Claims and underwriting can both benefit from leveraging AI. Everyone knows that reducing claims by writing better risk profiles COMBINED with managing those claims with an optimal mix of automation and technology to enhance claims decisions will lead to considerable competitive advantage. That requires the upskilling and reskilling of people combined with the right technologies.
Set a goal for AI-powered underwriting that reduces loss ratios by 10 to 15 percentage points COMBINED with digital claims transformation to reduce loss ratios by a further 5 percentage points. Ambitious insurers may aim even higher.
8) CREATE AN AI GOVERNANCE AND LEADERSHIP STRUCTURE
It is one thing to have an AI leader to evangelise and be in charge of how AI is deployed throughout the organisation. It is quite another thing to create a culture that focuses on data-driven decisions and actions. That requires a CEO that is familiar with IT leading the project.
- Motivate and get buy-in throughout the organisation
- Support teams and collaborative partners through the rough as well as the smooth.
- Deal with the ethical and regulatory aspects that may limit what a company can do
- Knows that this will take time, money, and commitment and will not be a short-term deliverable.
9) INVEST CONTINUALLY
Ambitious insurers that choose to be aggressive with AI should know that is not a decision to be made lightly. The move will have an impact for decades and require large budgets. They can, however, plan and deploy on a stage-by-stage basis and seek to make returns on investment for each stage so funding further stages.
The HBT article describes how CCC Intelligent Solutions has spent and continues to spend more than $ 100 million a year on AI and data. Its machine-learning models are based on more than a trillion dollars' worth of historical claims, billions of images, data on vehicle parts, repair shops, collision injuries, and regulations. I am sure the same may be said for Verosk, Solera, LexisNexis, Mitchell International et al.
Here's the thing- carriers can leverage this investment for a fraction of the cost especially as AI is itself making it less expensive to model events and predict outcomes.
Today there are many data, analytics, and AI service providers that can deliver the benefits of AI at a pro-rata lower cost to insurers. But, it still takes a continual and large investment as you add in operational, change management, and upskilling/reskilling costs.
10) ALWAYS SEEK MORE SOURCES OF DATA
Carriers generally collect and harness telematics data but how many collect and store GPS data? This is a rich source of insights to add to a data lake and enable better underwriting and claims management. Don't forget that if it comes from a policyholder's phone they must give permission for you to use it for the purposes you plan.
It is no good just collecting the data from your policyholders- you need data from the wider population if you are to predict more accurately, be selective on whom and what you cover. Practically real-time pricing data across vehicles new and old, parts and materials for home and motor, labour rates, and replacement costs for gadgets. That is if you want to manage to reserve effectively.
Data is often a nightmare- how many in underwriting and claims still rely on Excel? Try and get central IT to help manage data and when they find out it's Excel they will probably roll tgeor eyes and put your project at the bottom of the pile- into the notorious "pit of despair".
Luckily there are data and analytics consultants who will extract, and load data including Excel into data lakes and have the analytics platforms to transform the unstructured & structured data into usable and actionable insights and decisioning engines. It will likely take a long time, and will not be cheap but it will be effective. And if you want to be a top quintile insurer that not just survives and grows you will need to invest in this.
Google's recent announcement of its Insurance claim processing reference architecture | Google Cloud Blog is a major initiative to help carriers join all the dots though it does mean committing to a Google ecosystem. Amazon AWS and MS Azure can be expected to be on the same track.
The virtuous circle of more data, better models, more business, and more data is what will eventually make an insurer's application of AI powerful. New data will continue to flow into the company, and it will be used to improve estimate predictions and other functions. That will help insurers make better decisions, which will most likely bring insurers more business and more data.
"We also believe that AI—applied strategically and in large doses—will be critical to the success of almost every business in the future. Data is increasing at a rapid pace, and that’s not going to change. AI is a means of making sense of data at scale and of ensuring smart decisions throughout an organization. That’s not going to change either. Artificial intelligence is here to stay. Companies that apply it vigorously will dominate their industries over the next several decades. "
HBR
Here is a small selection of data sources insurers can leverage today
- CCC- featured in the HBR article; 50 billion miles’ worth of historical data through telematics and sensors in vehicles
- CoreLogic- manage property data for selling, financing, and protecting property
- Hazard Hub- property risk data
- Greater Than- a global database of vehicle/driver GPS and telematics data
- ICEYE- global flood earthquake and CAT damage data in near real-time
- KETTLE- house-by-house risk assessment across the USA
- LexisNexis- vehicle, ADAS, and home data
- McKenzie Intelligence Services Ltd wide range of data sources and data management
- Mitchell- Auto data
- SAFEHUB- building-specificS earthquake damage
- Synectics identity, financial, and fraud data
- Terrafirma property risk data
- Verisk- auto and property data
- WeatherNet- granular and near real-time weather data
- WhenFresh- wide range UK property data
Looking for AI solutions able to leverage this data?
Policy Admin, Claims Validation, and Triage
- Iotatach
- Omn:ius
- Open GI
- Sprout.ai
Claims Damage and Repair Estimation
- BDEO
- Be Valued
- CCC
- Claims Genius
- ClickIns
- LexisNexis
- Mitchell
- SLVRCD
- Solera/Audatex
- Symbility (CoreLogic)
- Tractable
- Upptec
- Value Checker
- Verisk
- Xtract360
Liability Assessment
- BAIL
Counter Fraud
- 360Retrieve
- BAE NetReveal
- FRISS
- Quantexa
- Shift
Payments
- Imburse
- Mastercard
- Stripe
And Claims Platforms?
Ecosystem Claims Management Platforms
- CoreLogic for property repair and restoration
- Verisk for property and auto repair, restoration, and total loss decisions
New Claimstech Management Platforms
- 360SiteView
- Claims Genius
- Claim Technology
- RightIndem
- Salesforce Industries (Insurance)
- Snapsheet
Further Reading
Despite progress in AI-powered claims handling, human judgment remains ‘key,’ Mitchell says
Claims automation: separating fact from fiction.
From Prediction to Transformation across Claims
AI and its related technologies will have a seismic impact on all aspects of the insurance industry, from distribution to underwriting and pricing to claims. Advanced technologies and data are already affecting distribution and underwriting, with policies being priced, purchased, and bound in near real time. An in-depth examination at what insurance may look like in 2030 highlights dramatic changes across the insurance value chain.